Undergrad Research Assistant
Language explination for self-Driving Scences
Abstract
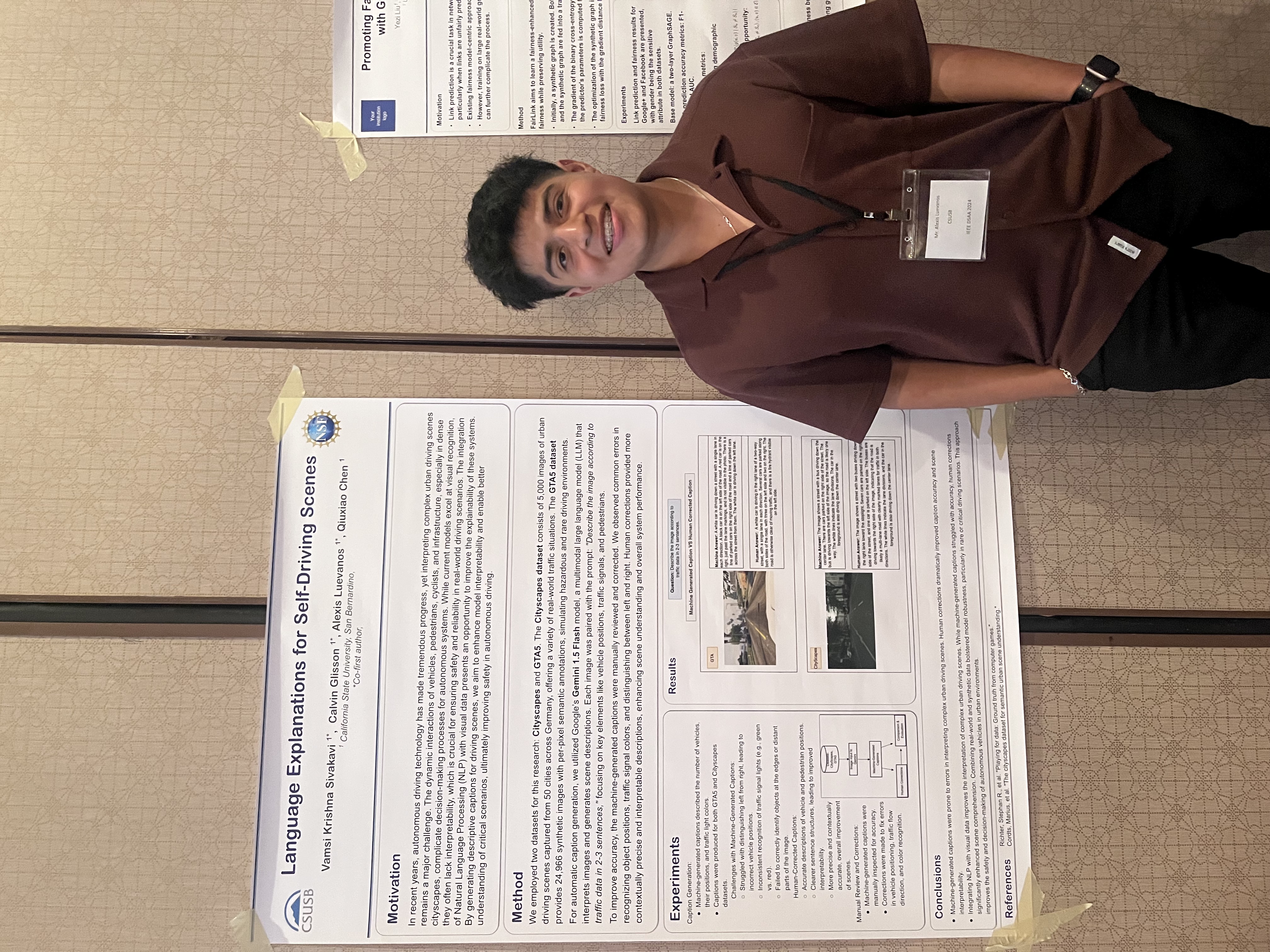
As part of my undergraduate research, I collaborated with a team to advance the integration of natural language processing (NLP) with visual data to enhance autonomous driving systems. This project addressed the challenge of interpreting complex urban environments, a significant limitation of current autonomous systems.
Methodology
- We utilized two datasets: the real-world Cityscapes dataset and the synthetic GTA5 dataset, containing images of urban driving scenes.
- Google's Gemini 1.5 Flash model was employed to generate captions for each dataset, focusing on traffic-related details like vehicle positioning, traffic signals, and pedestrian activity.
- Human-corrected captions were created to improve the accuracy and contextual relevance of the machine-generated descriptions.
Duties and Contributions
- Generated automated descriptions of urban scenes using AI models and developed traffic-focused captions for datasets.
- Performed manual reviews and corrections to refine machine-generated captions for accuracy and contextual clarity.
- Co-authored a research paper presented at the IEEE DSAA Student Forum, detailing our findings and dataset contributions.
Outcomes and Success
- Participated in the IEEE DSAA Student Forum. Learn more here.
- Featured on the CSU News for achievements at the IEEE DSAA Conference. Read more here.
- Introduced two novel datasets integrating visual and text-based data for self-driving applications.
- Enhanced understanding of how AI can interpret urban environments and inform autonomous systems.
- Presented findings at an international conference, showcasing the innovative approach and practical impact.